Driven by advancements in technology and the broadening of application areas, the data annotation market is undergoing a transformative shift. In the last year, the market size of data annotation grew from $1.28 billion to $1.7 billion, with projections to reach $5.27 billion by 2028 [ResearchandMarkets].
As AI continues to be integrated across industries, the demand for high-quality, reliable training data keeps growing. To meet this demand, many businesses are turning to AI-powered data labeling solutions. However, relying solely on automation for specialized, high-precision training data raises critical questions. Can automated annotation truly meet the complex needs of businesses today? Through this blog, let’s figure out whether automated data annotation solutions are sufficient or if a human-in-the-loop approach is necessary for creating dependable AI model training datasets.
Trends Raising the Need for Automated Data Annotation in 2024
Techniques like Programmatic Labeling, Active Learning, and Transfer Learning are accelerating the adoption of automated annotation across data-driven industries. Additionally, several emerging factors make people more inclined toward automated data labeling, such as:
1. Rise in Unstructured Data
Millions of terabytes of data are generated every day on the web, and the majority of them are unstructured (coming from disparate sources in diverse formats). As IDC forecasted, by 2025, the global data volume is expected to reach 175 zettabytes (ZB), with 90% of that data being unstructured. Manually processing and labeling such a sheer amount of unstructured data is impractical for businesses, leading to reliance on automated solutions.
2. Expansion of Autonomous Systems
The adoption of autonomous vehicles, robots, and systems is increasing in industries like healthcare, agriculture, and logistics for real-time object & event detection and decision-making. However, to operate efficiently in complex environments, these systems require accurate labeling of visual data (drone images, surveillance and camera recordings, LiDAR sensor data, etc). Manual labeling cannot meet the data demands of these systems at the required scale, speed, and precision, making automated annotation a critical component in their development and deployment.
3. Increasing Adoption of Large Language Models
Advanced large language models like GPT-4, LaMDA, and Llama2 have transformed NLP capabilities, from language translation to dialogue systems, allowing businesses to create more sophisticated applications. As the NLP market heads toward a $68.1 billion valuation by 2028 [MarketsandMarkets], the demand for large-scale annotated data continues to rise. This demand makes automated data labeling solutions critical to support the development of more advanced NLP applications.
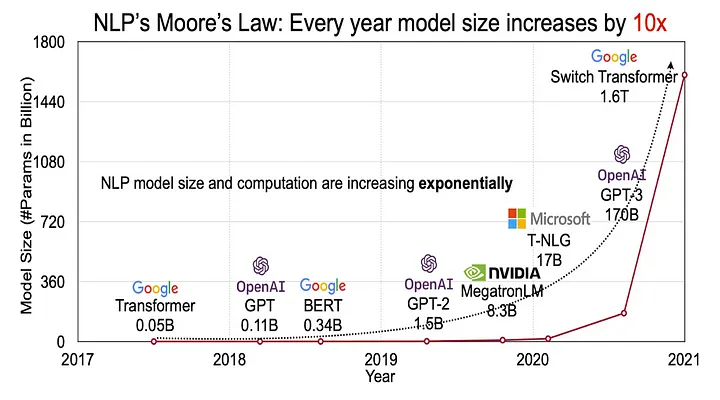
4. Need for Multimodal AI Systems
Industries like healthcare, retail, and entertainment are increasingly adopting multimodal AI for tasks such as medical imaging, virtual try-ons, and personalized content recommendations. To function correctly, these applications require accurately labeled data across diverse data types (such as text, images, and videos). The speed and scalability required for annotating multimodal datasets cannot be achieved through manual methods. Utilizing automated data annotation tools, these models can be trained faster, reducing the time-to-market for AI-driven products.
Factors Restraining the Adoption of Data Labeling Automation
For businesses, while automation seems like a promising approach for large-scale AI/ML data annotation, it can have negative outcomes if implemented without human supervision. This is primarily because automated tools for data labeling have their own shortcomings, such as:
● Ethical Challenges
One of the most concerning aspects associated with AI data labeling tools is bias. If the training data fed to annotated systems is biased, it will get amplified in the data annotated by such tools. Decisions based on biased data can be ineffective for businesses, leading to discriminatory outcomes, especially in sensitive domains like healthcare and finance.
To ensure AI model fairness and ethical outcomes, it is critical that subject matter experts identify and mitigate bias from training data. Utilizing their domain expertise, they can identify patterns that may discriminate against specific groups (e.g., racial, gender, or socioeconomic bias) and make the required changes in the training instructions to ensure fair representation.
● Data Security Concerns
While efficient and scalable, automated data annotation tools may raise questions about data privacy and security. In sectors like healthcare, finance, and legal, to develop a specialized app, you have to feed sensitive information for labeling to the automated tool. What if that tool compromises data security protocols, exposing your confidential data to cyber attacks and breaches?
While human supervision cannot completely eliminate this risk, it can be decreased to a significant level to avoid possible breaches. By anonymizing personally identifiable information, establishing data governance policies, implementing data encryption & role-based access, and ensuring that sensitive data doesn’t remain on labeling system for a long period after use, human administrators can minimize the chances of unauthorized data access.
● Lack of Contextual Understanding for Subjective Cases
AI-powered data labeling solutions struggle with grasping context in several scenarios as their understanding is limited to their training data. If something falls outside their rule-based algorithms, they may mislabel it without understanding the deeper meaning. Subject matter experts are critical for context-aware annotations especially where complex terminologies, logic, or interpretation are required.
For instance, in retail, the word “clearance” could refer to selling off stock at a discount, but in logistics, it could mean customs clearance. Without expert input, AI could misinterpret the term and mislabel crucial retail data.
● Inability to Handle Edge Cases
Automated data annotation models rely on large, diverse datasets for AI model training. If unusual scenarios (edge cases) are poorly represented in the training data, the model will struggle to identify and label them correctly.
For example, imagine an image labeling tool trained mostly on pictures of vehicles taken in clear daylight. When the tool encounters images taken in foggy conditions, it may fail to accurately recognize vehicles because its training data didn’t have enough foggy images. In these cases, subject matter experts, with their experience, can step in to correctly identify and label objects, even in challenging situations like low-resolution or foggy images.
Crowdsourcing and Outsourcing Models – Viable Alternatives to AI-Powered Data Labeling Solutions
So, while automation brings efficiency, the accuracy and contextual relevance in data labeling still demand human intelligence. To leverage the capabilities of both, you can:
● Outsource Data Annotation Services to Professionals
Instead of solely relying on automated data labeling solutions, you can partner with a reliable third-party provider for annotation projects. Experienced in large-scale data labeling, these providers have a dedicated team of subject matter experts and access to advanced automated tools to ensure both accuracy and efficiency. Utilizing multi-tier QA processes and an inter-annotator agreement approach, they ensure quality and consistency across all annotations while complying with data security and industry regulations.
Real-world example: How outsourcing data labeling helped a municipal agency in infrastructure maintenance and urban planning
The municipal agency wanted to train an AI system to detect certain objects on the street (such as litter, debris, potholes, and manhole covers). They had a large dataset of 3000 images to be annotated for AI system’s training and outsourced this task to a reliable data labeling company. The team’s expertise played a critical role, particularly in efficiently handling edge cases, such as challenging images with low visibility or complex backgrounds. The annotated dataset improved the accuracy of the client’s object detection algorithm by 45% and reduced the operational cost involved with manual inspections by 30%.
● Utilize Crowdsourcing Platforms for Large-Scale Data Annotation
Distributing data labeling tasks among a large group of annotators through a crowdsourcing platform can be another cost-efficient way for large-scale projects. These platforms have experienced annotators who can label data in your stipulated timeframe, adhering to the labeling guidelines. However, one potential challenge that can arise is keeping the labeling consistent, as different annotators may interpret the data in slightly different ways.
This challenge can be overcome by providing detailed labeling instructions to all annotators and ensuring everyone follows them to maintain uniformity in the annotated dataset. Additionally, supervisors or experienced annotators can review and validate the work submitted by the crowd to ensure consistency.
Key Takeaway: Automation and Human Supervision Go Hand in Hand for Superior Data Labeling
Undoubtedly, the future belongs to AI and automation, but not without human supervision. To create dependable AI/ML systems and mitigate challenges like bias, ethical usage, and model transparency, automated annotation needs to be validated by subject matter experts. Depending upon your project needs, budget constraints, and scalability requirements, you can decide which approach will suit you to integrate human oversight in labeling workflow: develop an in-house review team, partner with outsourcing experts, or tap into crowdsourcing resources. The key is finding the right balance to ensure both efficiency and accuracy for AI/ML data annotation.